Product Analytics Overview
Someone recently asked me about my comfort with data in the context of product management. And while I think they may have been asking about my SQL proficiency, I prefer to talk about data in the broader context within an organization.
I've been fortunate to work in some data-literate organizations with extremely mature data stacks from the early days. Unfortunately, I also know how rare this is. Companies usually start out fresh, dare I say, clean. Here's the more typical scenario: A company gets started > everyone can access everything, and nothing is too muddy, yet > a product goes live, a lot of people join, a lot more data is generated > nobody can figure anything out > a company magically soon becomes worth $1B > they invest heavily in cleaning up their stack, who has access to what, etc.
Anyway, this post is mainly in the context of product management for those who, like myself, aren't extremely proficient but at least know my way around and who to cozy up to for help when needed. And while the scenario above will remain true for a while, I'm excited about the evolution that will improve this for many companies. Thanks to companies like Definite, it'll be easier to have more mature, cleaner data stacks from the early days, and more people who are less technical will be able to analyze them. It may even become more common to have verticalized (out of the box) analytics tools that generate dashboards, alerts, etc., specific to your product category and role. But until then, the most important thing for PMs is to understand the big picture and the types of metrics and analysis you'll need to define, measure, and act on.
Product Analytics
Here' the big pic. A company has a bunch of data. There's a very messy middle. And then you have the ability to do analysis.
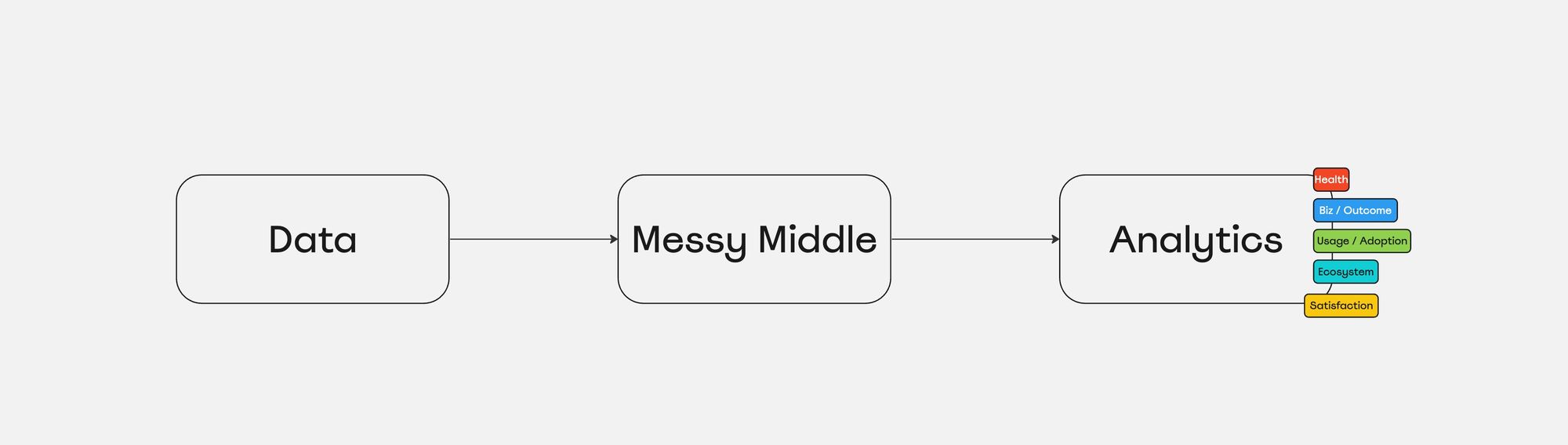
I love Shreya's product metrics categories, although I collapse adoption and usage usually.
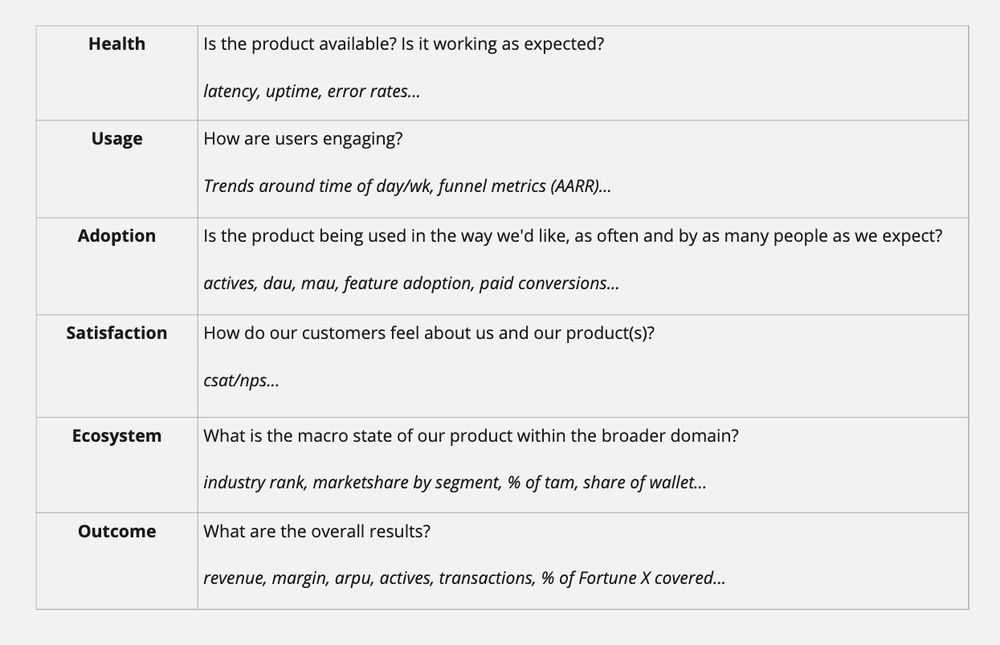
Let's get more granular.
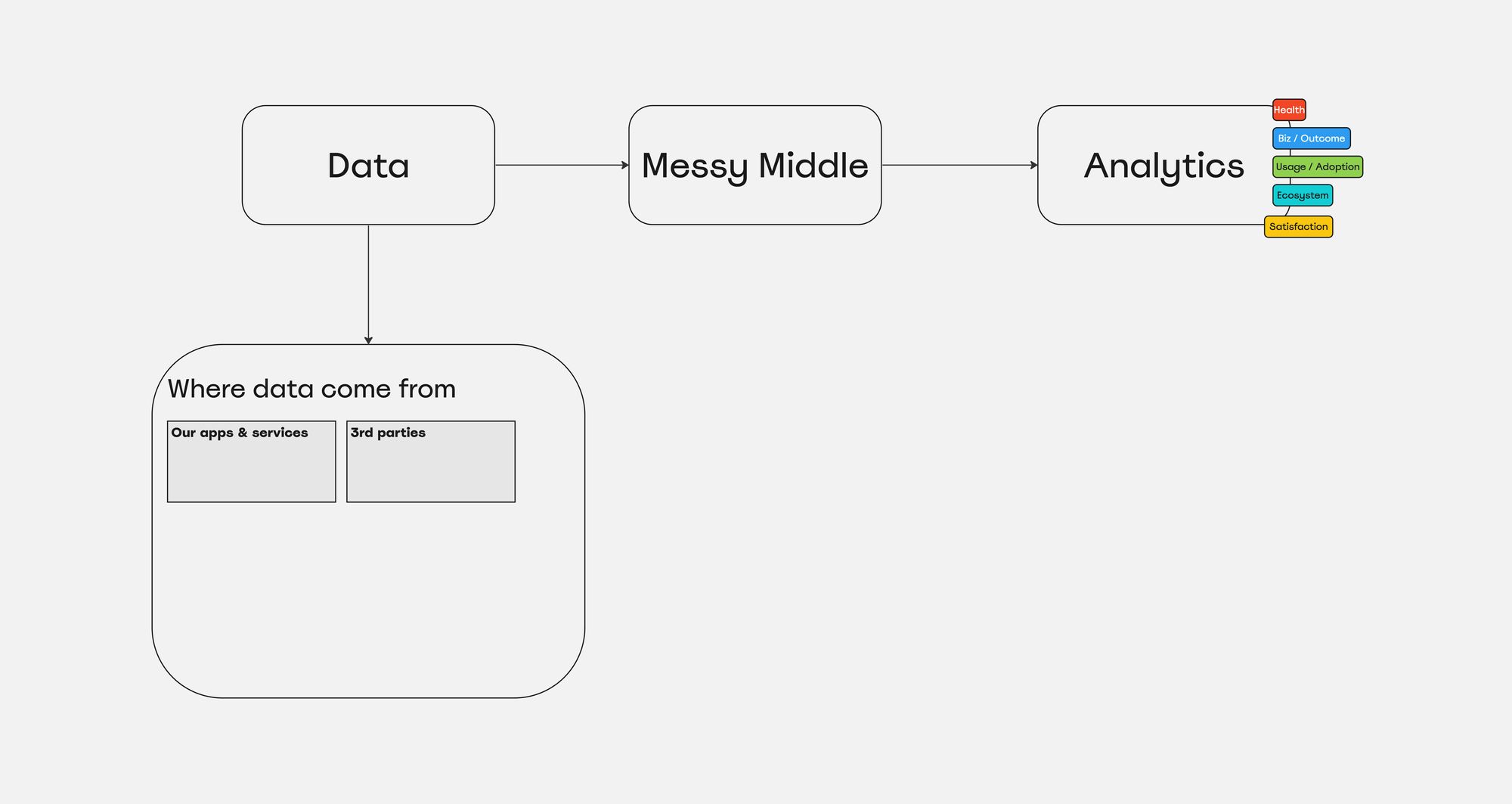
Data actually come from a lot of places. Some initiated by users, some by various systems. Some comes from your own systems, many comes from 3rd parties.
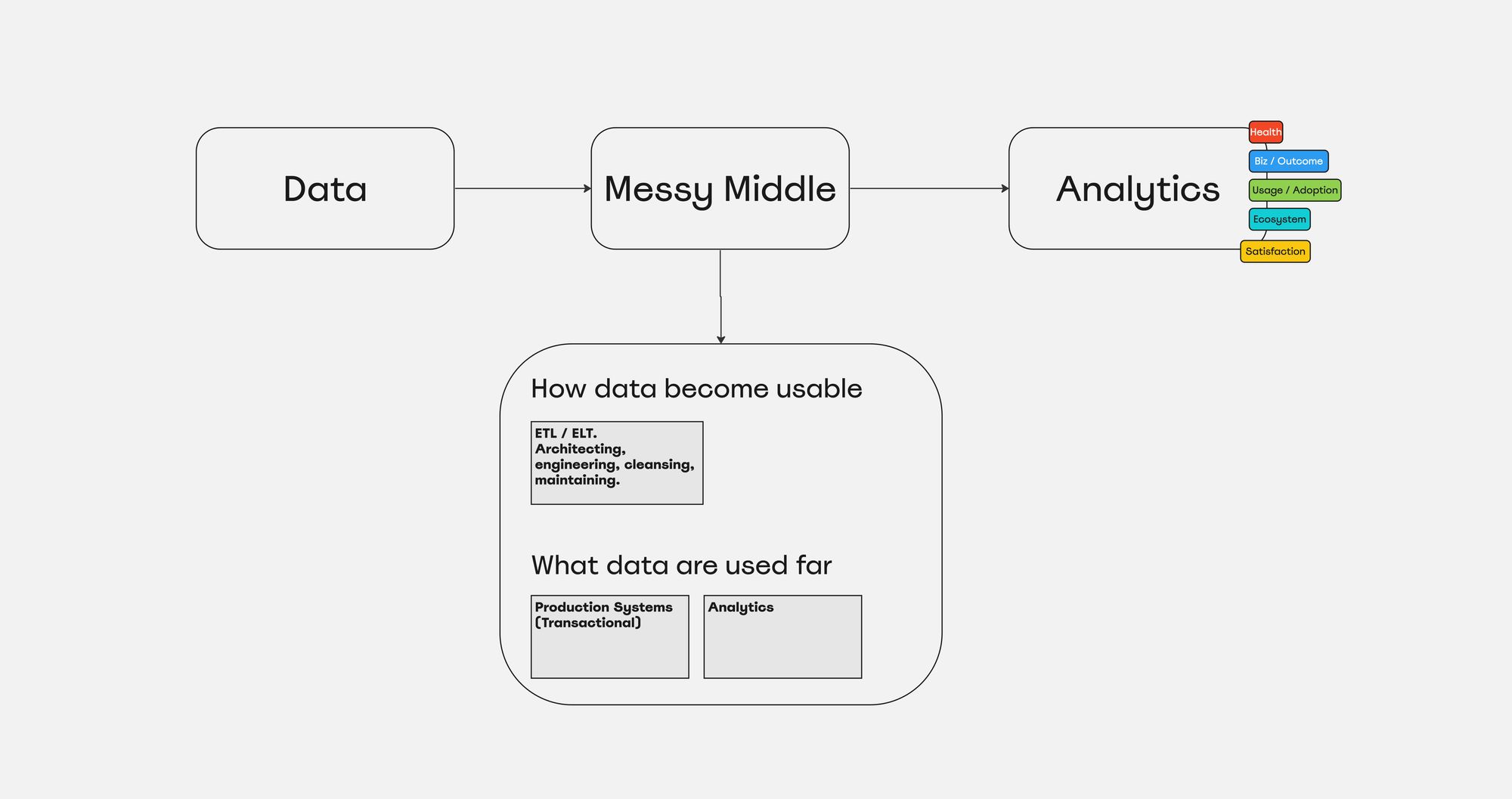
Data are used for analytics as well as for powering production systems. Data are rarely useful for analytics without a very messy middle. A lot of people take this for granted. It takes highly competent people and effort to architect this properly, and to maintain with time. This is often where company culture and founding team DNA shine.
The more third parties you depend on, the tougher this can be. In the case of Blispay, we built a credit card product with a direct integration to TSYS. To get the data from their mainframe system, our team had to pull off a monumental effort to essentially create a bunch of batch jobs that extracted, transformed, and loaded data into our warehouse. It was painstaking... mapping every field in what they call a 'master file' to fields of our own, accounting for constant breaks and changes. This is the stuff most companies don't bother with because they don't value the effort or simply don't have the technical capability to pull off. This is also the type of stuff common in sectors like fintech, that others who work at tech companies that own most of their stack can take for granted.
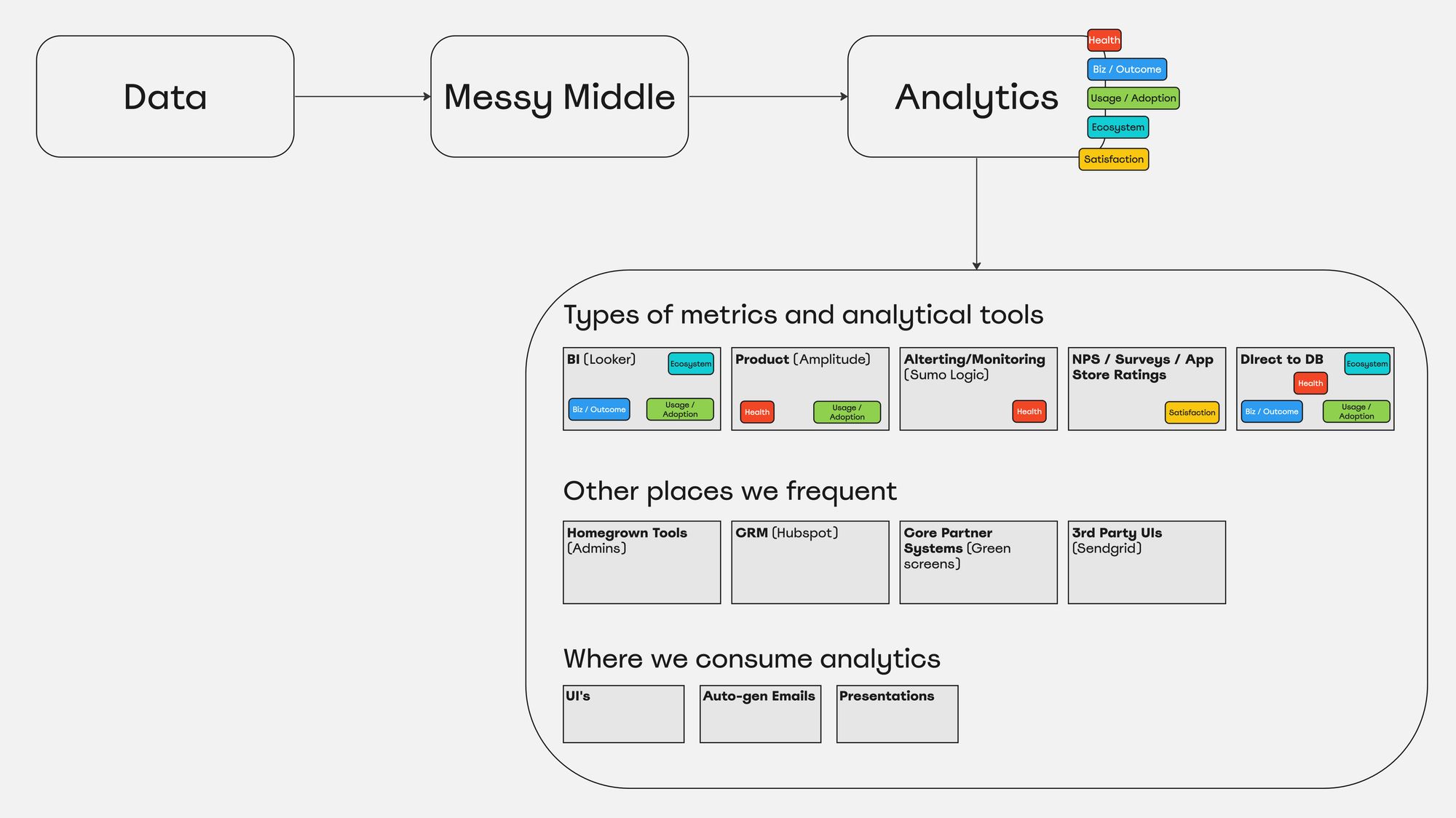
As a product manager, you'll likely live in these tools as much as you live in Jira and Slack. They can be overwhelming and difficult to navigate if you weren't involved in the actual definition and creation of the various metrics, dashboards, or reports. It's nice if you can create your own (even if you need to work with someone on a data team). While it can be painful, it's the only way to know exactly you're looking at. The bigger the company, the harder it can be to access various tools or data. You may not get access to a logging tool like Sumo Logic or to surveys or ratings that marketing "owns." Just do your best to define the fewest number of reports (and ideally create dashboards) that give you the broadest view into the things you care most about, whether that be an entire product or just an individual feature you're responsible for rolling out.
Examples
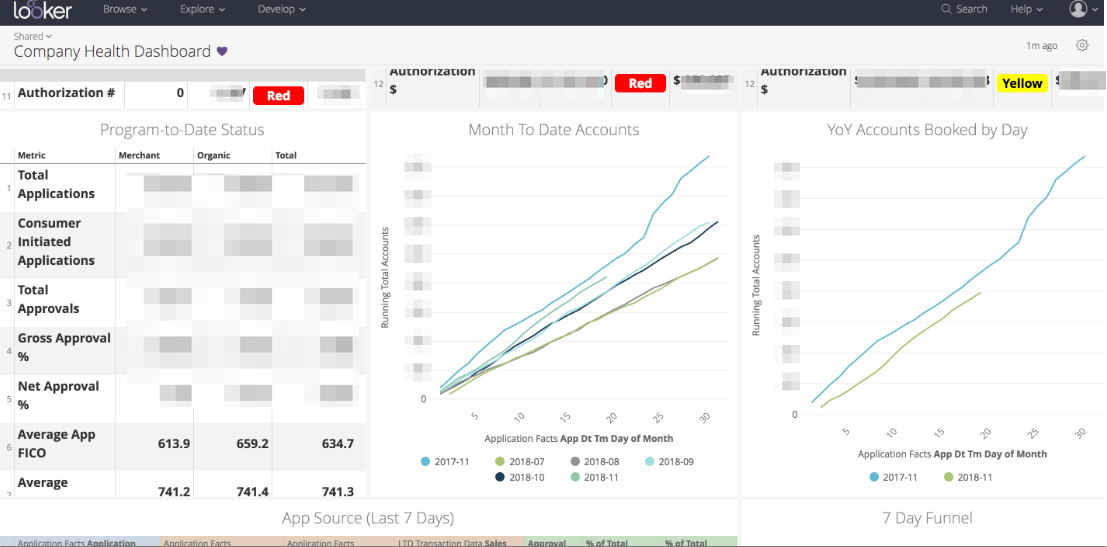
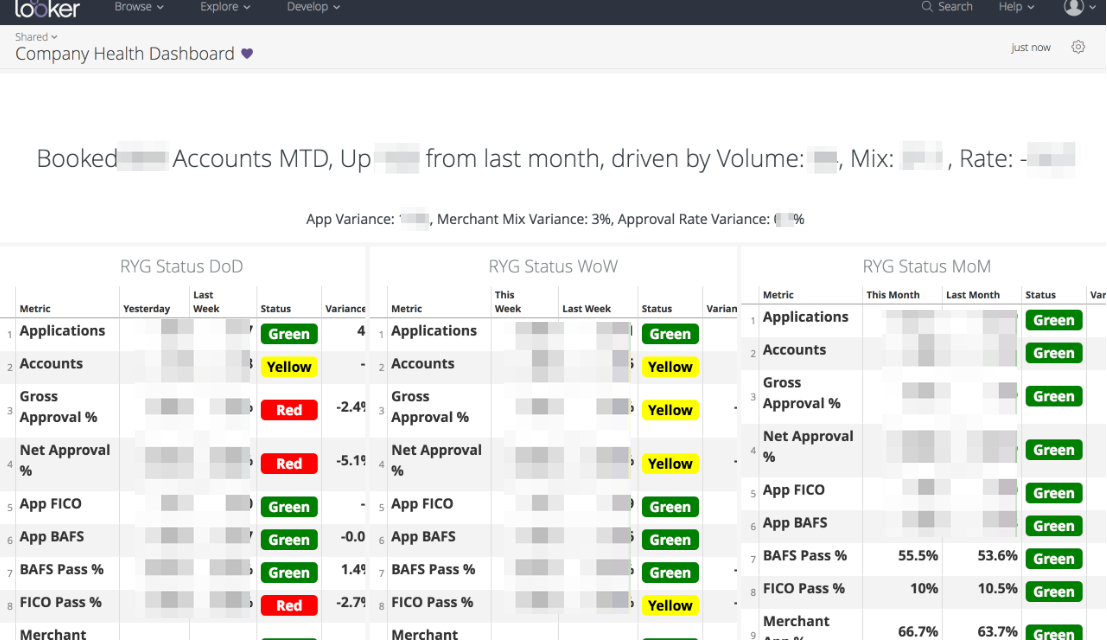
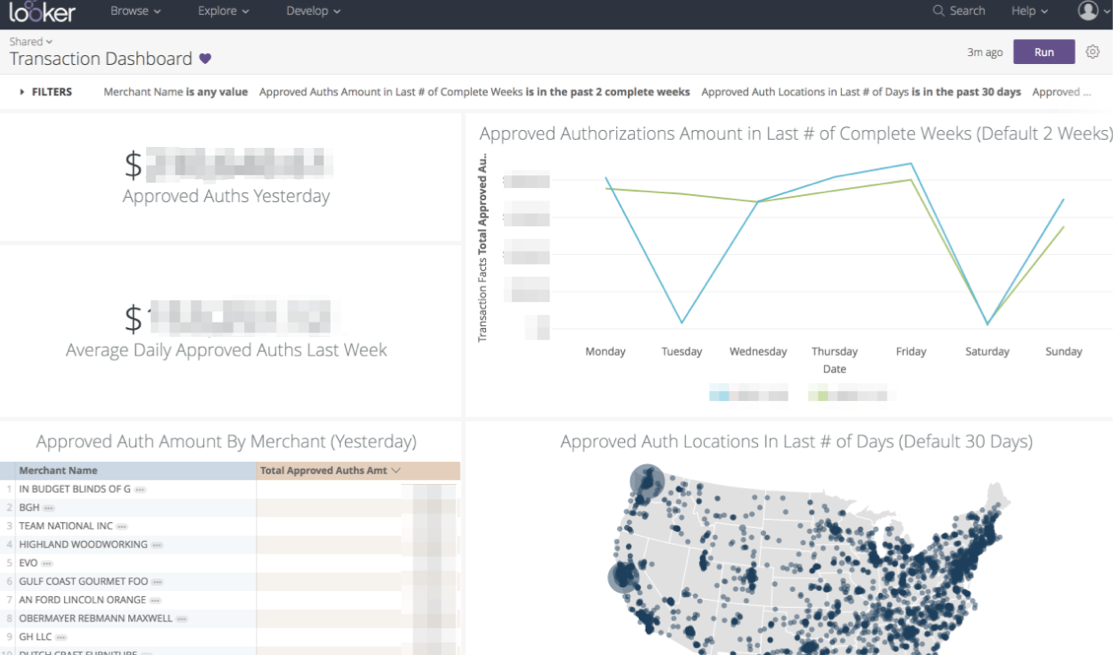
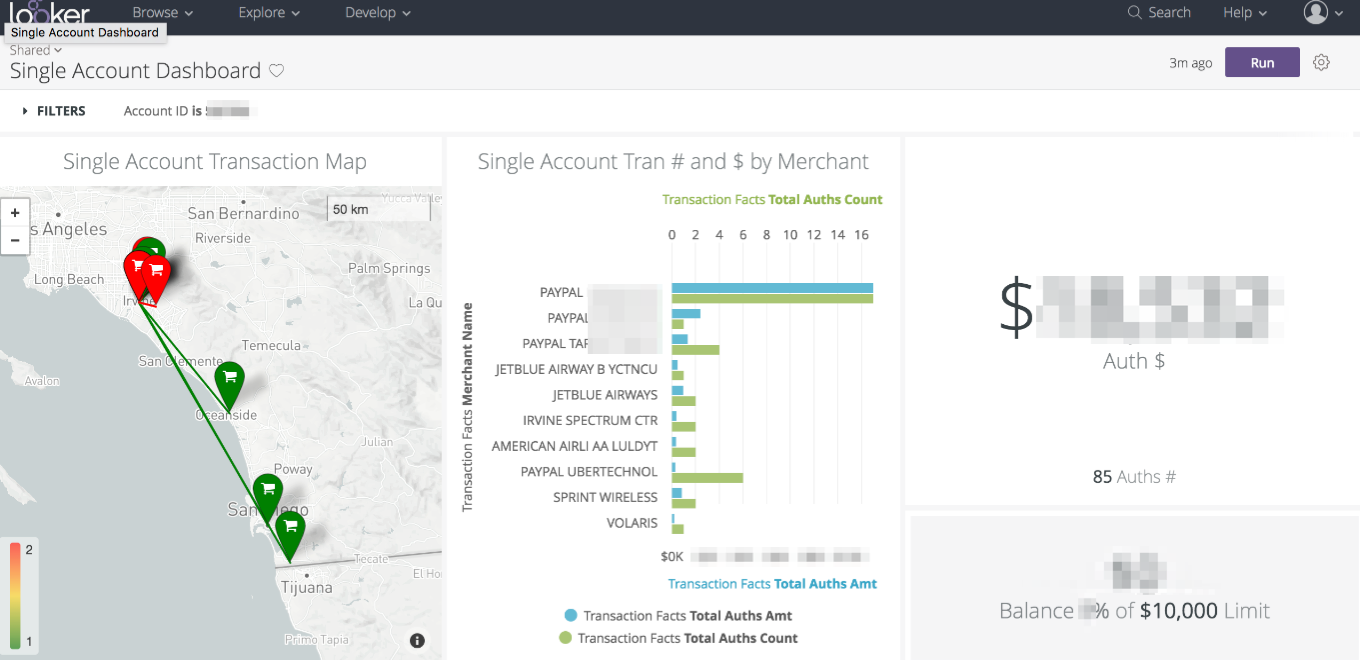
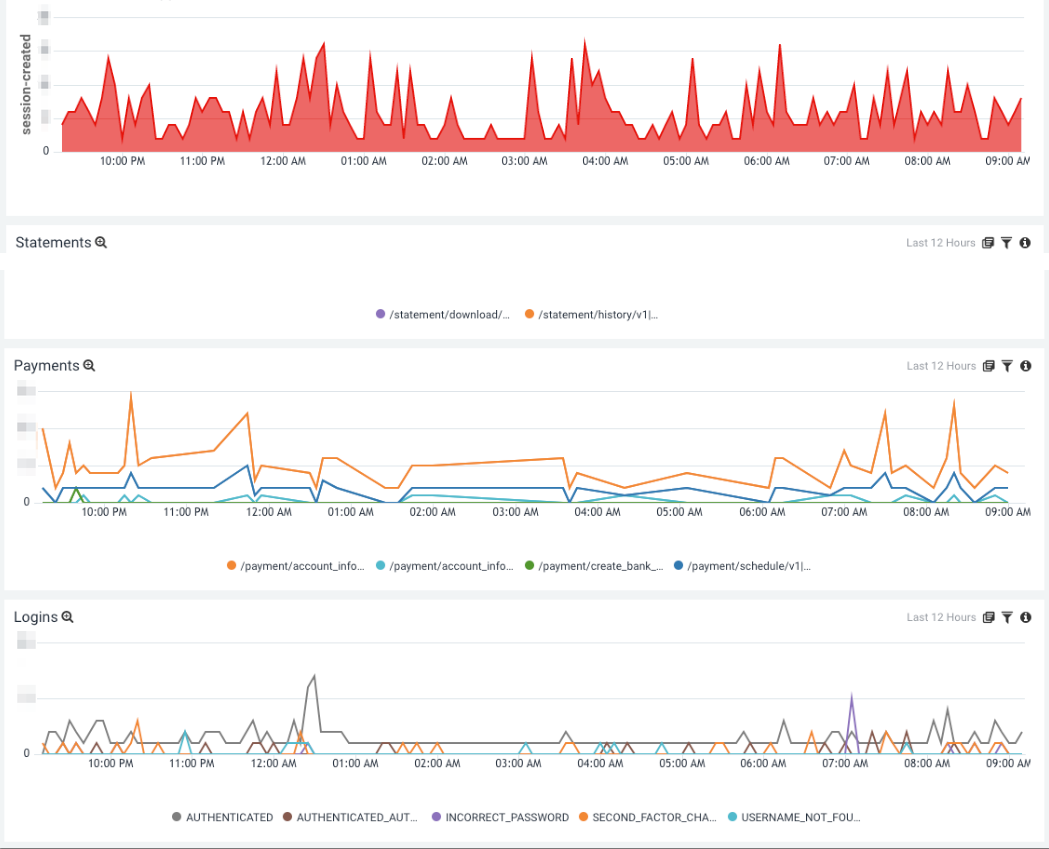